Fashion Machine Learning Applications and Examples
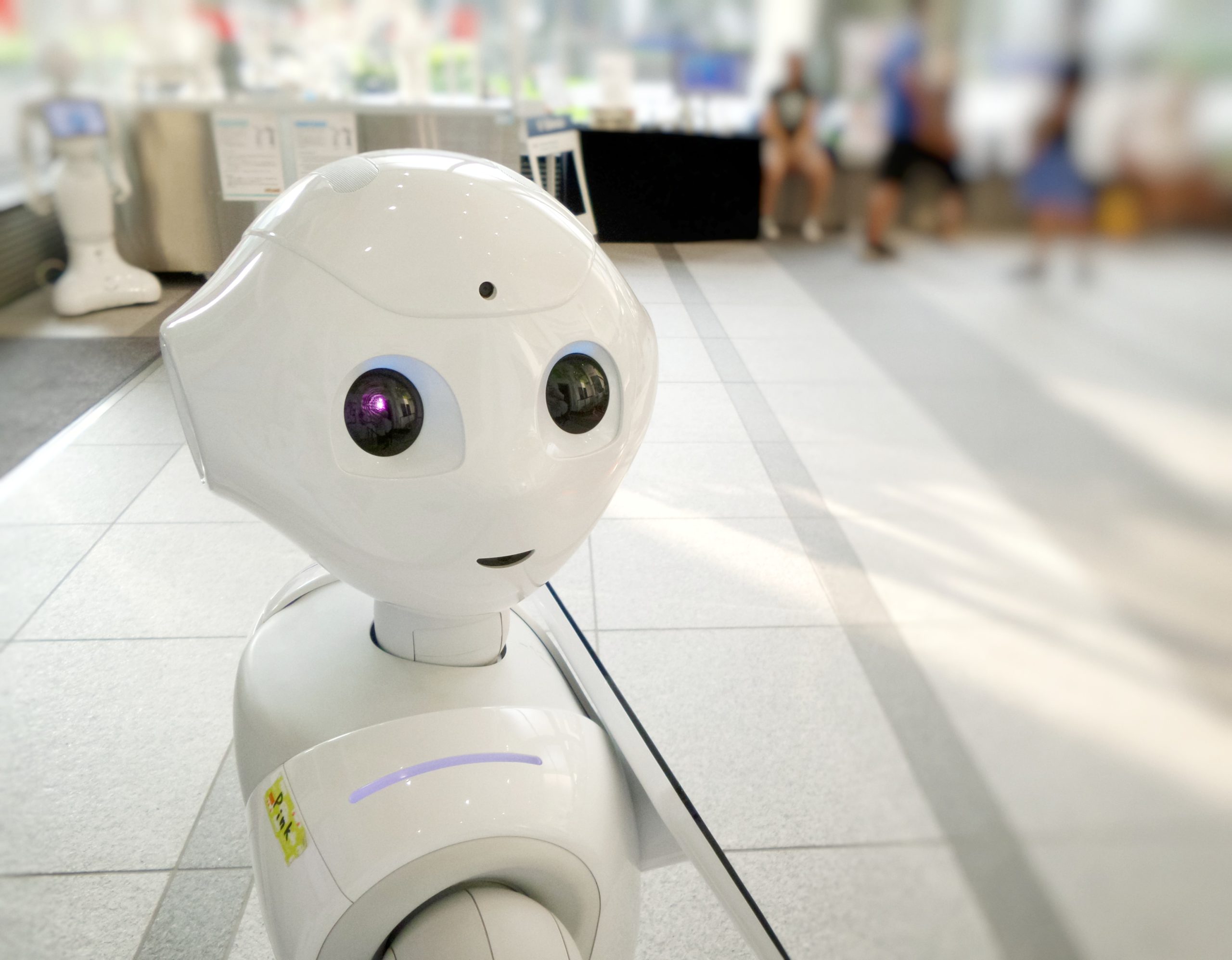
In 2018, Reuters reported that the Spanish apparel retailer Zara was incorporating artificial intelligence (AI) into its business strategy and supply chain as a way to keep up with and beat its competitors. Coincidence or not, but the following year, Zara’s parent company Inditex posted strong profit growth. And even though 2020 — the year the COVID-19 pandemic shut down the world — was tough for everyone, so far, Inditex sales have topped pre-pandemic levels.
Of course, Zara isn’t the only fashion retailer leveraging AI and machine learning (ML) to stay ahead of the curve. Tommy Hilfiger, H&M, and Dior, as well as many other brands, some of them fast-fashion and others haute couture, are doing the same. Most recently, the clothing and accessories retailer GAP acquired the AI startup Context-Based 4 (CB4), which it hopes will help improve its customer experience by enhancing predictive analytics and demand forecasting.
According to a 2021 study by Juniper Research, 96% of surveyed retail executives plan to invest in AI in the near future. What’s even more interesting is that an NTT DATA and Oxford Economics survey found that 40% of executives think that AI is crucial to business success and that a failure to implement the technology will end up costing them their customers and employees, as well as hurt their bottom line.
Seeing these numbers, many retailers may feel compelled to explore how they can apply AI and ML to their business operations — and with good reason. From trend forecasting to personalised recommendations to automated customer services, the use cases for fashion machine learning are many and varied. Here are just some of them.
Designing and Manufacturing
Today, it is not unusual for a fast-fashion brand to have around 52 “micro-seasons” in a year. This means that, on average, customers can expect their favourite brand to drop one new collection a week.
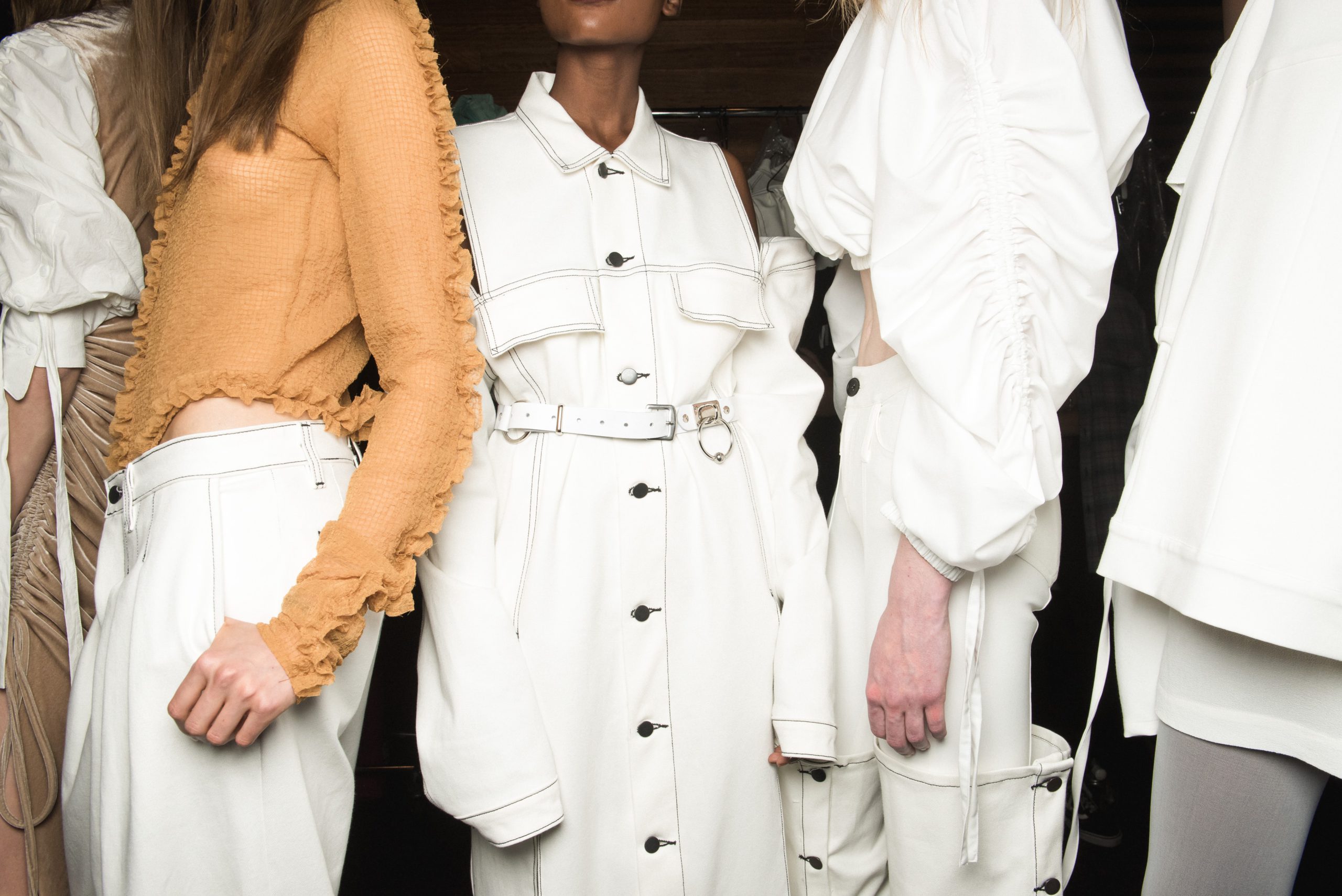
Coming up with this many new apparel designs and then actually making them in a timely manner can be a real challenge for designers and manufacturers involved — especially considering that most fashion items can take anywhere between 6 months and a year to develop. And for retailers, the trend-driven nature of fashion can make estimating customer demand for their new line of products tricky, to say the least.
So it comes as no surprise that an increasing number of fashion companies are turning to AI and machine learning for help with the process:
- AI-driven demand forecasting has been proven to reduce forecasting errors by up to 50%.
- “AI designers” can analyse popular styles and design elements, such as cut and fabric, as well as their past retail performance and popularity on social media, to create completely new designs from scratch.
- AI-enhanced garment manufacturing leads to improved efficiency and time-to-shelf due to automated quality control and defect detection.
Retail Strategy
One of the worst things that can happen to a fashion retailer is ending up with unsold stock. Unfortunately, this is a far more common occurrence than most people may realise.
In 2018 H&M’s lengthy supply chain lead times coupled with the brand’s inability to understand consumer preferences left it with $4 billion of unsold inventory. To overcome this issue, the multinational fashion retailer turned to machine learning.
Having analysed store purchase history at one of its stores in Stockholm, the company realised that its inventory stocking strategy was severely lacking. For example, in this particular store, H&M was stocking basics for men, women, and children — even though its customer base was mostly women. By removing its menswear line and instead focusing on stocking more trendy items for women, the store was able to reduce its stock by 40%. No doubt H&M replicated this same experiment across its other stores, too. With intelligent buying and merchandising planning, you can do the same.
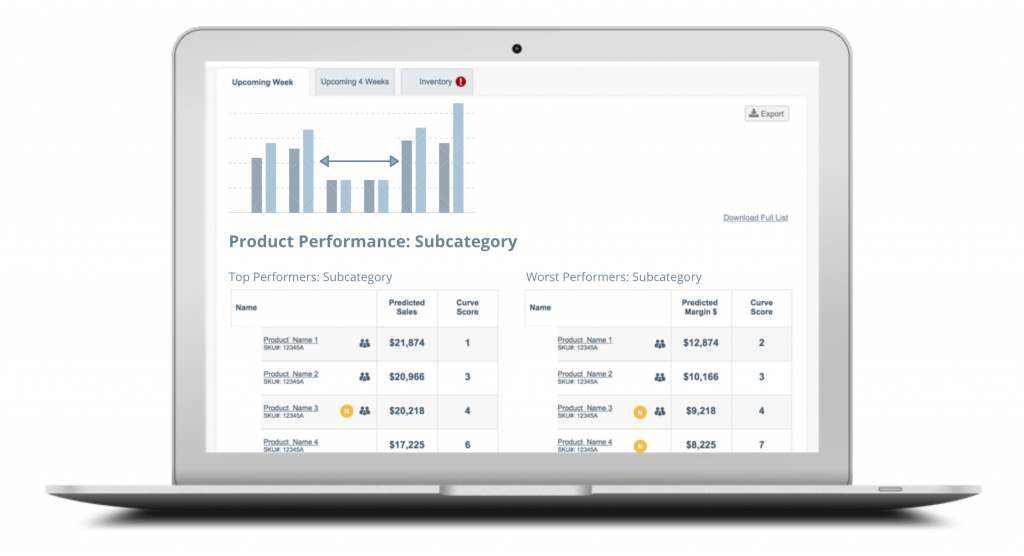
Besides informing assortment, AI and ML can also help brands come up with the best product and pricing strategies based on what their competitors are doing.
Supply Chain Management
ML and AI can also make the supply chain more efficient, agile, and sustainable.
For instance, by highlighting the best and worst performers in real-time, AI and ML allow brands to stop the production of items that no one wants and increase the production of garments that are trending in real-time. This can seriously reduce the likelihood that a brand will end up with dead stock.
AI and ML can also improve shipping times. Take Amazon’s “anticipatory shipping,” which was patented in 2013, as an example. By utilising predictive analytics tools and Amazon customers’ data, the tech giant can pre-ship products a customer is going to order (but hasn’t already) to nearby warehouses. Then, when a customer actually purchases the item, they can receive it much faster than if it was coming from a traditional fulfilment centre.
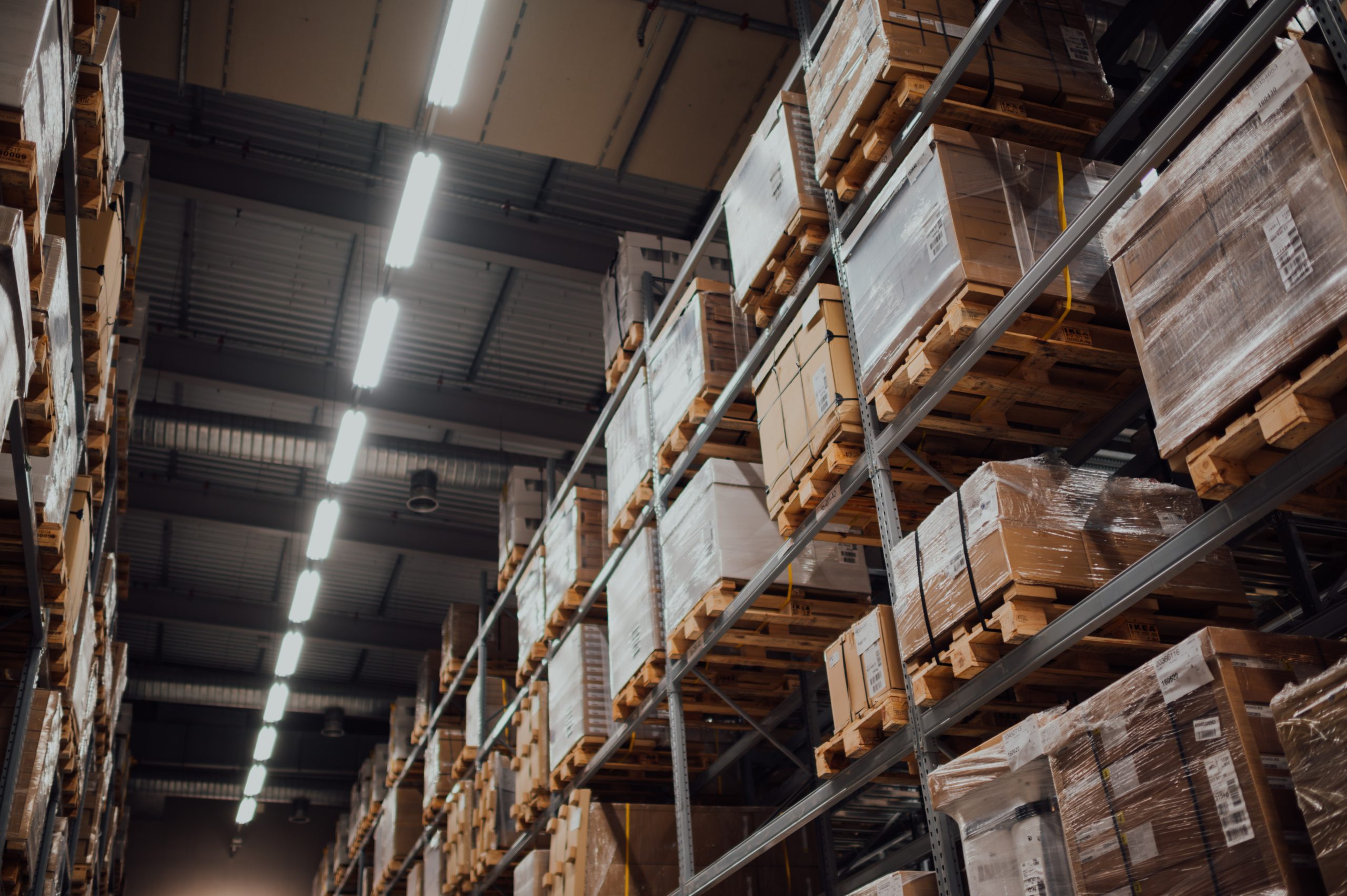
Some brands are also using AI to determine suppliers, modes of transport, and delivery routes that are the most sustainable.
Product Discovery
The internet has provided customers with access to thousands of online stores, and in consequence, millions of products. However, as the paradox of choice theory teaches us, more is not always better.
One study found that the more shoppers search on an online clothes store, the more weary they grow, and the more likely they are to take a break from online shopping. Crucially, these breaks can last up to a week and may not always result in a sale.
For fashion retailers, then, helping customers navigate their product catalogue and surfacing the most relevant results is non-negotiable.
One way AI and ML technologies can make product discovery easier is by augmenting search. This could be through automating product tagging, a feature that enriches products with detailed and specific attributes (such as their colour, fabric, or print) and allows customers to find items that match precisely what they’re looking for.
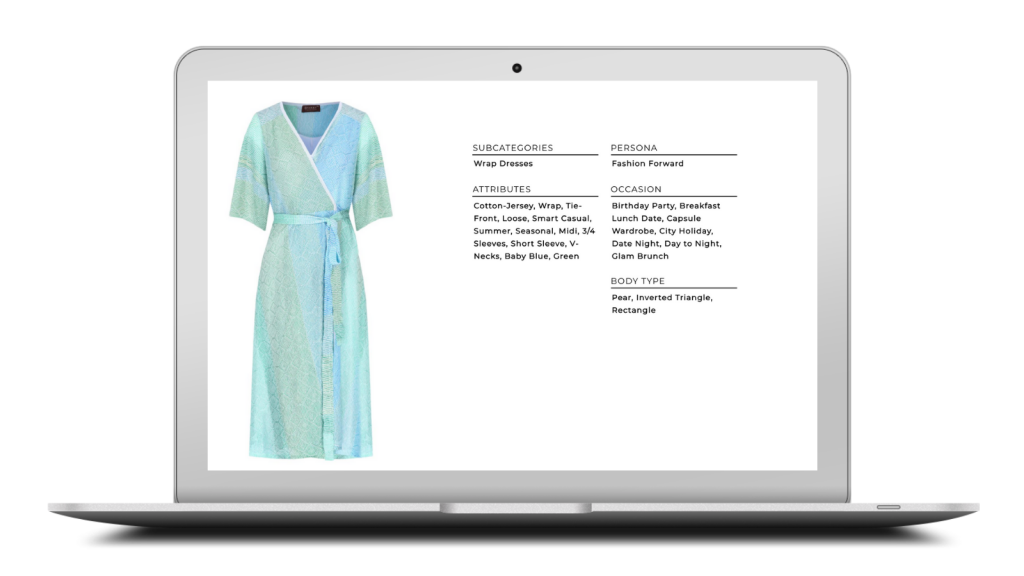
Or, it could be through natural language search, which means that shoppers can search for items using the language they speak in their everyday lives.
For example, AI and ML are integral to Amazon’s “contexts of use” system, which helps Amazon understand the intent of a customer’s search query. Thanks to this system, Amazon can know whether a person is looking for “waterproof shoes” because they’re planning on going hiking or because they need to keep their feet dry when walking to the subway on their way to work, and can then surface relevant results.
For customers that may struggle to put into words what they want, visual search can be invaluable. AI-enabled visual search can identify all the products in a photo uploaded by a customer and then show items from the retailer’s product catalogue that best match them.
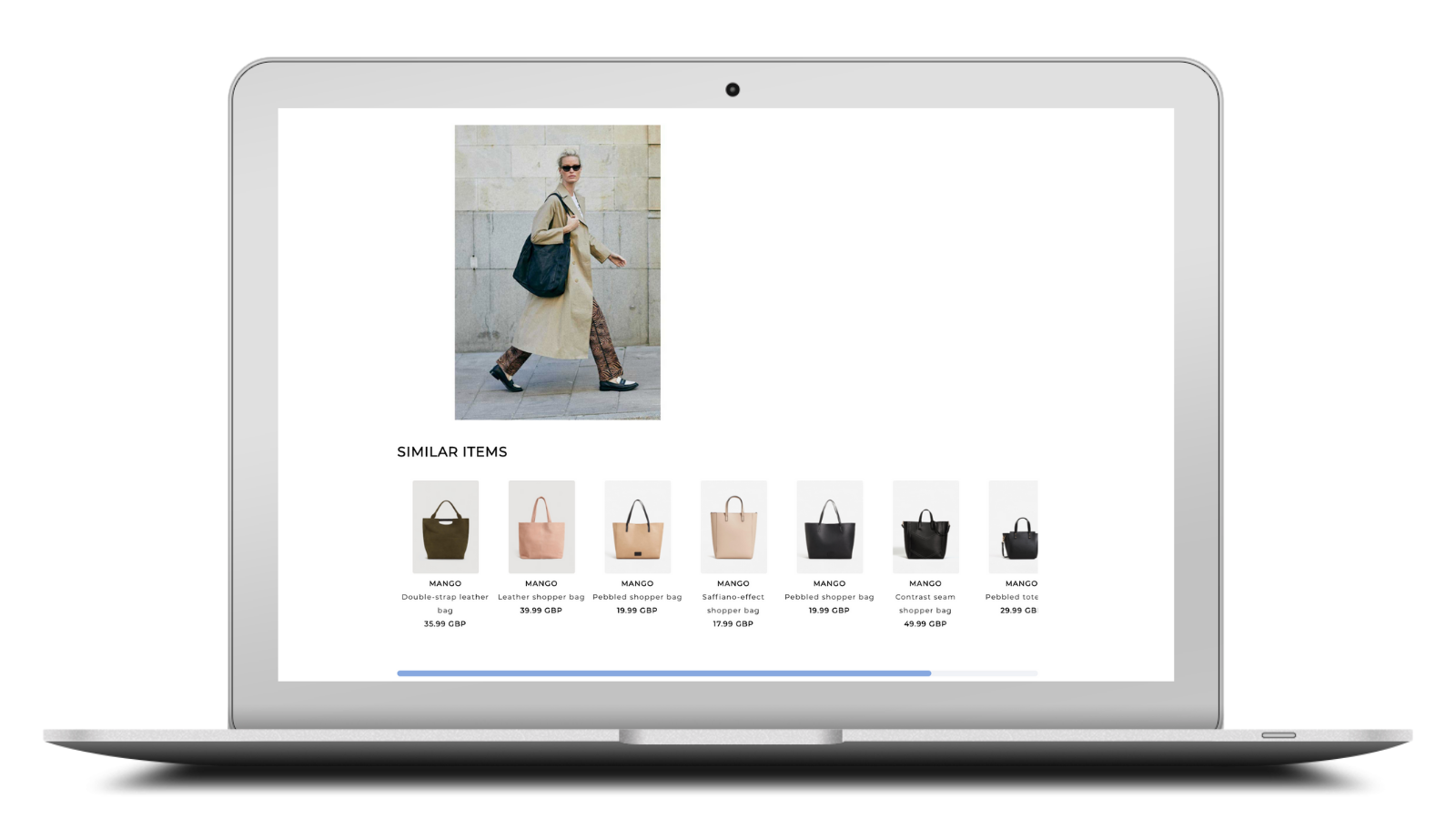
Fashion retailers can also use AI and ML to personalise recommendations on product pages.
When customers click on a product on Lane Crawford’s webpage, they are shown not only the item they’re interested in but also garments that are visually similar to it. That way, even if the customer doesn’t love the item they clicked on, or it’s out of stock, their product discovery journey doesn’t end — they have other, highly relevant options to explore.
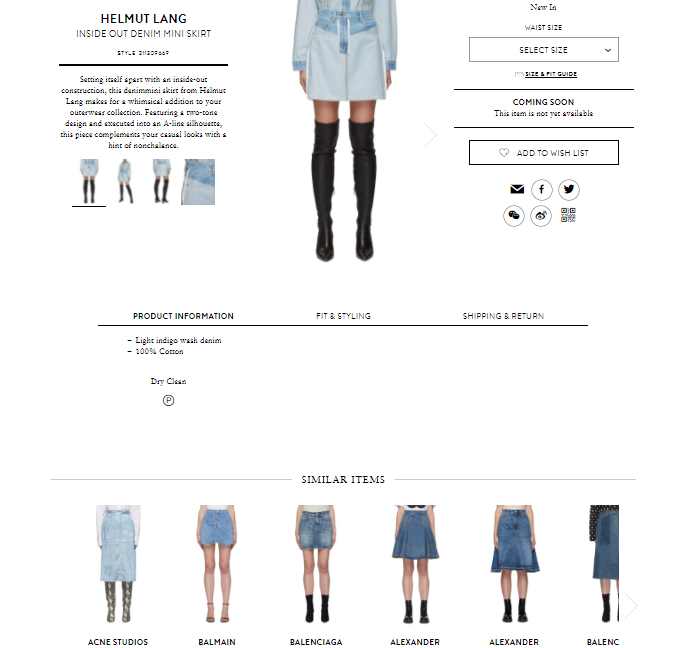
For shoppers that may really like an item but struggle to come up with ways to style it, Lane Crawford offers “Complete the Look” suggestions. Not only can this inspire the customer to buy the item they originally looked at, but it can also motivate them to purchase items that go well with it, thus increasing their overall basket size.
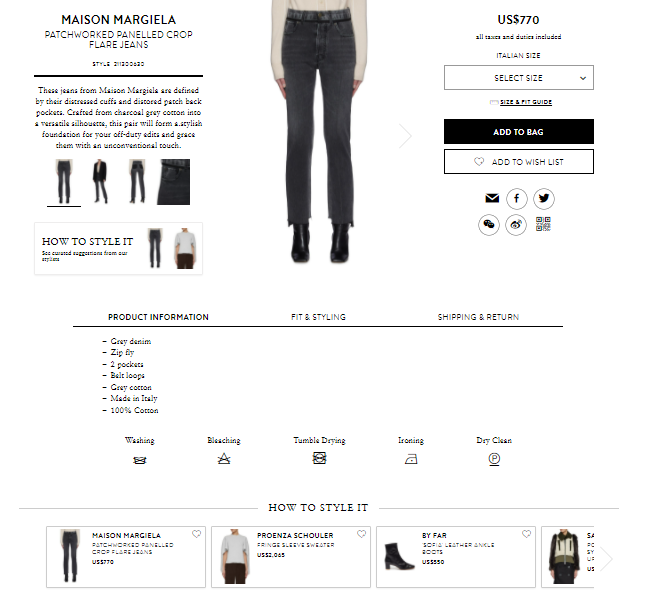
Tommy Hilfiger takes this a step further by giving customers styling options based on different occasions. And Stich Fix, everyone’s favourite personal styling service, has developed an algorithm that generates outfit suggestions based on a customer’s own unique style.
Physical stores can also offer customers personalised recommendations, and not necessarily through sales associates. Rather, retailers can install smart mirrors that recommend outfits based on the style of the clothes that the customer is currently wearing. Customers could also use these smart mirrors to scan an item they come across to see how they could style it with other garments in the store. In the fitting room, if an item does not fit as expected, customers could potentially use smart mirrors to get alternative suggestions and have the items brought to them directly.
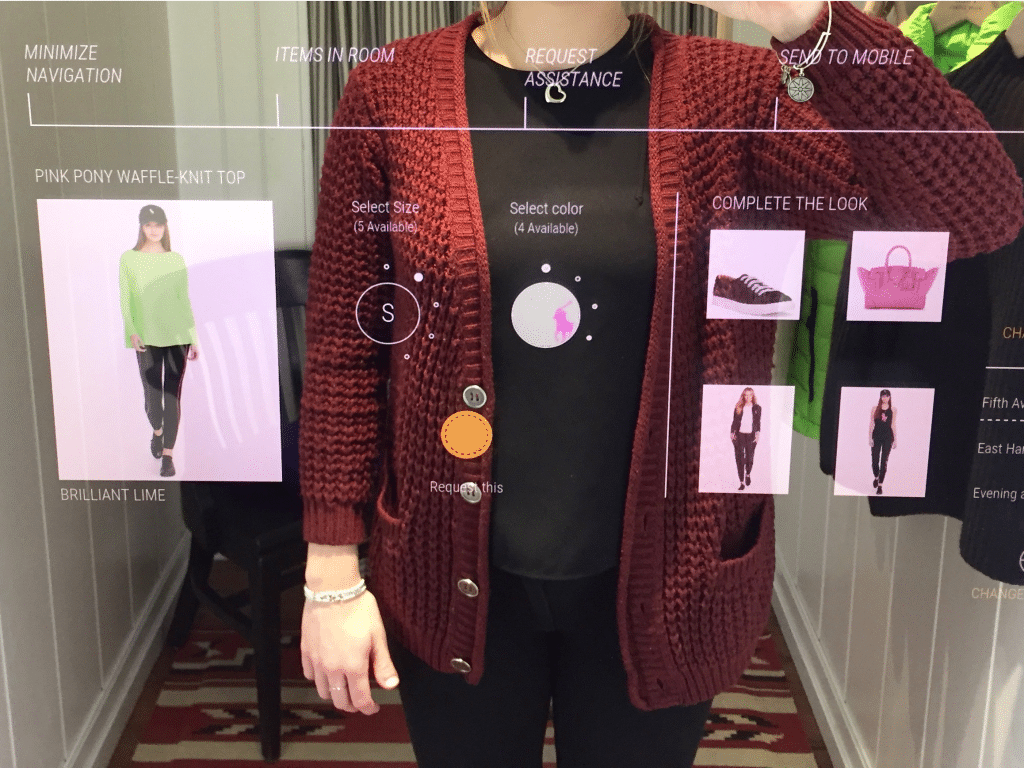
However, retailers don’t have to wait for customers to visit their site or store to show them personalised product recommendations. Instead, they can use personalised promotional and retargeting campaigns to help them complete a look or bring their attention to items they are most likely to engage with.
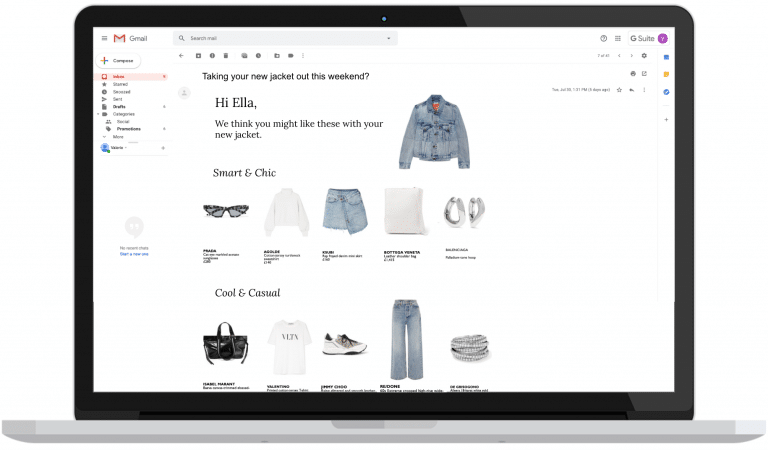
Nordstrom, for example, used ML to come up with an “inferred scoring” system based on data it collects from its customers (such as clickstreams, purchase data, and email campaigns) to show them items they most likely want or need. This technique improved Nordstrom’s marketing campaign effectiveness and grew its email-to-dollar conversion by a whopping 25%.
Fashion Machine Customer Service
A customer representative may not always be around to help, but by “employing” AI chatbots, retailers can be certain that their customers will always be taken care of.
In 2017, Dior launched “Dior Insider,” an AI chatbot that customers can enable through Facebook Messenger, and that can answer Dior product queries and share the latest Dior news.
That AI chatbots can be incredibly powerful is illustrated by Alibaba’s “AliMe” chatbot. During “Single’s Day” in 2017, AliMe answered 9 million queries and generated 56.7 billion personal recommendation shopping lists for shoppers. And in 2018, AliMe was upgraded to provide:
- Pre-sales promotion (product recommendations and intelligent shopping reminders to finish transactions).
- During sales and post-sales services (changing addresses and appointing logistic vendors).
- Intelligent shopping assistant (AliMe can read a customer’s emotions to alert human customer support agents).
It was thanks to AliMe that Alibaba made an impressive $31 billion on Single’s Day in 2018,
Chatbots are not limited to a retailer’s website or Facebook. They can also be integrated into instant messaging apps like WhatsApp, WeChat, and iMessage.
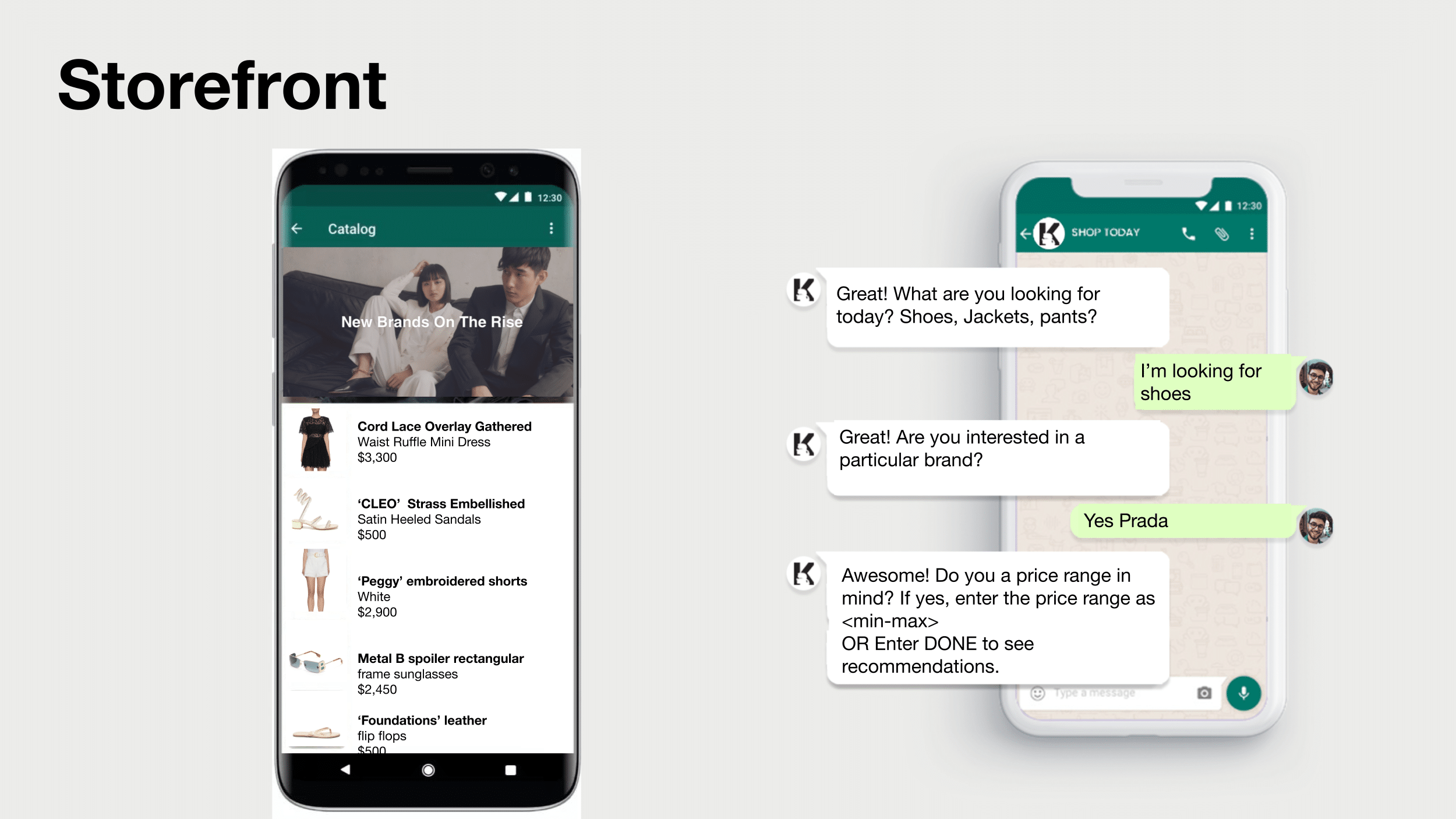
Fashion Machine Learning: Where to Go From Here
All the big fashion brands are jumping on the AI and ML bandwagon. However, there is no reason why smaller brands can’t integrate the technology into their operations, either.
For more examples of how fashion businesses can use AI, check out our comprehensive whitepaper on the topic.
Ready to start using AI and ML? Whether you’re looking to personalise customer experiences or take advantage of advanced buying and merchandising reports, we can help.
Book a free consultation